SUMMARY: A session exploring how real-life research may aid decision-making and guideline development and complement RCT for better assessment of interventions.
Bias, both in randomised controlled trials (RCT) and Real-world evidence (RWE) is important to minimize, as pointed out by Dr Giorgio Walter Canonica (Milan, Italy). RCTs, on one hand might target a very narrow treatment group to mitigate confounding factors, whereas in RWEs, subpopulation results might get lost in the heterogeneity of the real world. While evidence of efficacy is the strongest with RCT, the evidence of effectiveness, i.e. how well a treatment works in practice in a broader population, is better shown with RWE1. And thus, they complement each other, and both are needed.
Better use of real-world data
Aiming to raise the profile of real-life research, the Respiratory Effectiveness Group (REG) was formed. Together with EACCI (the European Academy of Allergy and Clinical Immunology), certain quality standards have been implemented. One point of action is to establish more registries for more systematic capture of real-world data2.
The REG and EAACI also organized a workshop in March this year to discuss integration of RWE and EBM (Evidence Based Medicine) into next generation guidelines, in which regulatory bodies such as the FDA and EMA participated, as well as patient organizations. A second workshop in October will finalize the outcomes of the first workshop.
mHealth – getting more health data from mobile devices
The WHO (world health organization) defines mHealth as medical and public health practice supported by mobile devices, (e.g. mobile phones or personal digital assistants)3. With the huge collection of data, big data processing and artificial intelligence (AI) algorithms can be used for future evidence generation4, says Dr Hilary Pinnock (Edinburgh, UK). Still, only a minority of mHealth is embedded in routine healthcare5, although an increase has been seen from 2010 and accelerated by the COVID pandemic. While traditional healthcare data collects data at visits, mHealth has the potential to capture so much more data over the course of an illness and/or a lifetime. EAACI lists a number of areas, e.g. epidemiology, health economics, public health, where mHealth can be of help.
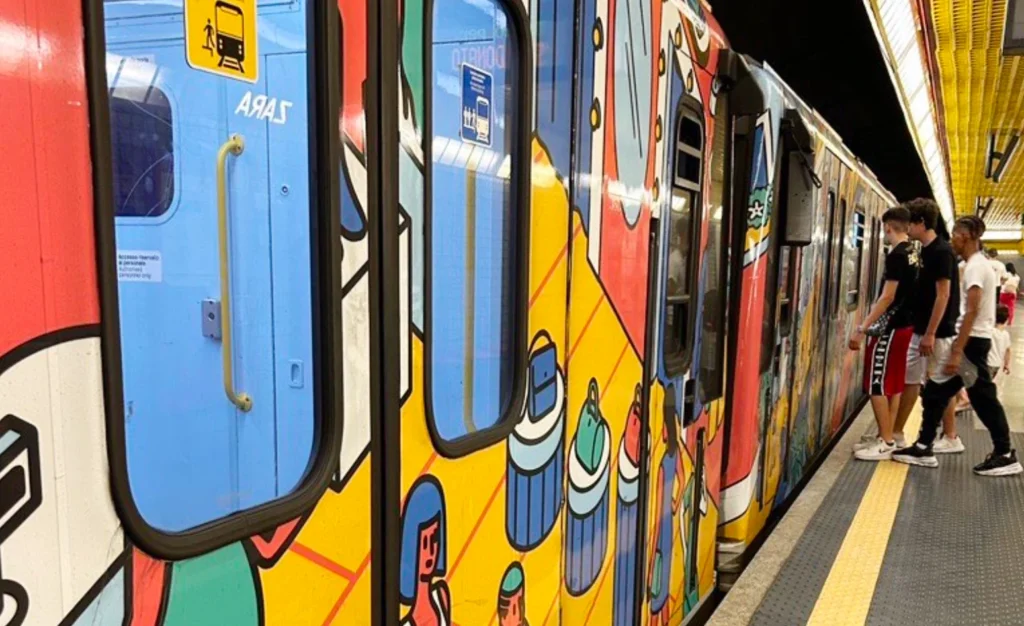
Do mHealth data represent everyone in the real world?
There is a risk that patient tools might attract a specific “type” of patient. In a study to demonstrate pollen and wildfire impact on asthma symptoms, predominantly white, male colleague students with high income signed up – however women over 40 were more likely to keep reporting data. Out of 7593 enrolled, only 64 and 37 respectively, logged persistent data throughout the study period.6 So what happens, if we use biased limited data to train AI?
Use of automated feedback, like wearable devices may provide data that is more generalizable. Integration of these systems into routine practice is also a task for policy makers and administrators. We have a long way to go, concludes Dr Pinnock, before we are generating data evidence well from mobile devices and mHealth tools.
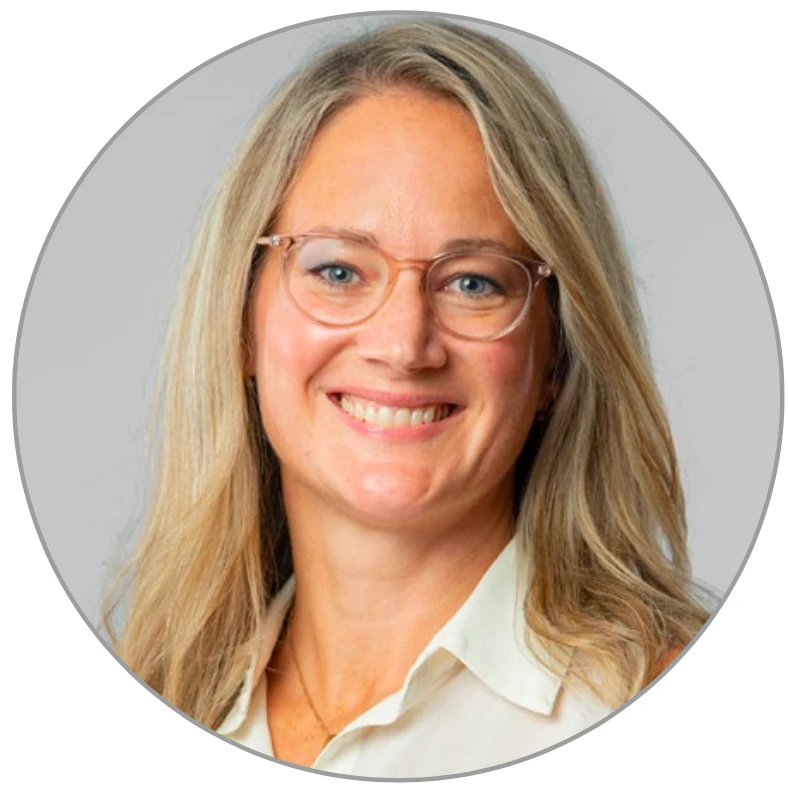
Christina Eliasson
Nordic Product manager
References:
- Paoletti G, Pepys J, Casini M, et al. Biologics in severe asthma: the role of real-world evidence from registries. Eur Respir Rev. 2022;31(164):210278. Published 2022 Jun 7. doi:10.1183/16000617.0278-2021
- Di Bona, D., Paoletti, G., Chu, D.K., Pepys, J., Macchia, L., Heffler, E. and Canonica, G.W. (2021), Allergen immunotherapy for respiratory allergy: Quality appraisal of observational comparative effectiveness studies using the REal Life Evidence AssessmeNt Tool. An EAACI methodology committee analysis. Clin Transl Allergy, 11: e12033. https://doi.org/10.1002/clt2.12033
- WHO Global Observatory for eHealth. (2011). mHealth: new horizons for health through mobile technologies: second global survey on eHealth. World Health Organization. https://apps.who.int/iris/handle/10665/44607
- World Health Assembly, 71. (2018). mHealth: use of appropriate digital technologies for public health: report by the Director-General. World Health Organization. https://apps. who.int/iris/handle/10665/276430
- World Health Organization. (2016). Global diffusion of eHealth: making universal health coverage achievable: report of the third global survey on eHealth. World Health Organization. https://apps.who.int/iris/handle/10665/252529.
- Chan YY, Wang P, Rogers L, et al. The Asthma Mobile Health Study, a large-scale clinical observational study using ResearchKit. Nat Biotechnol. 2017;35(4):354-362. doi:10.1038/nbt.3826
ID 345-2023-MARK /Sept 2023